

The waste container (Ebin) consists of two divisions, one for the bio-degradable waste and This project includes a cost-effective bin for small-scale
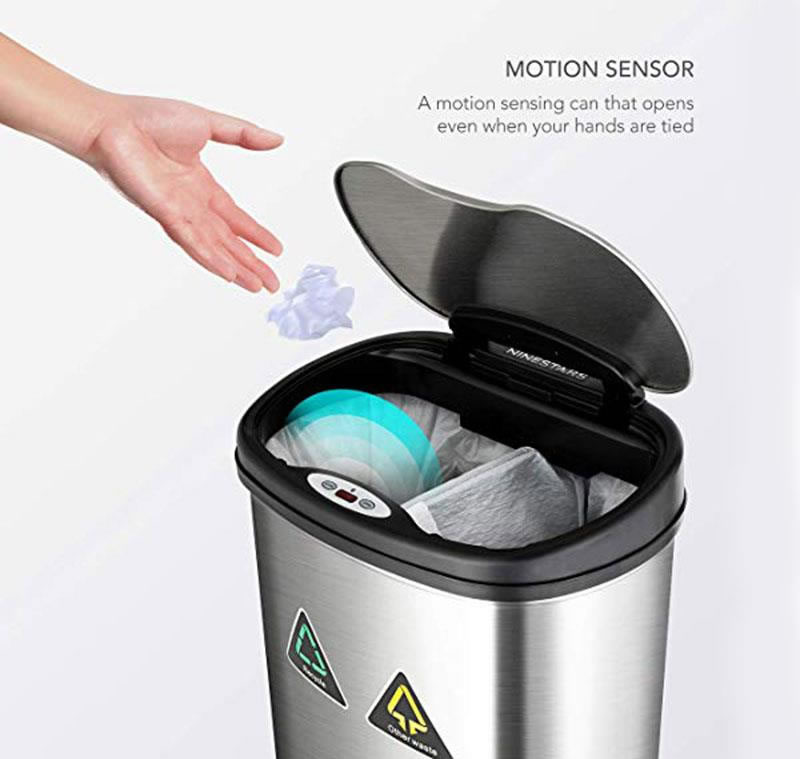
Indian roads, sewages, even obsoleteĪreas are plundered with plastic bottles, polythene bags, litters,Įtc. Waste is creating issues but also the biowaste due to the lack of The race is struggling to collect the waste and get Waste that the ways for collecting and disposing of it are now out The world has accumulated an enormous amount of The system proposed in this study is intended to be implemented in a rural area, where it can potentially solve the recyclable waste separation problem. To deliver the capacity monitoring feature, the uploaded trash bin capacity information is displayed on the mobile application in the form of a bar level developed in the MIT App Inventor for the user to quickly take action if required. The capacity and GPS information are uploaded to Firebase Database via theESP8266 Wi-Fi module. Several Internet of Things hardware, such as ultrasonic sensors for measuring trash bin capacity and GPS for locating trash bin coordinates, are implemented to provide capacity monitoring controlled by Arduino Uno. The performance of the YOLO model was evaluated to measure its accuracy, which was 91% under an optimal computing environment and 75% when deployed in Raspberry Pi. The classification result rotates the trash bin lid and reveals the correct trash bin compartment for the user to throw away trash. This study describes the development of a smart trash bin that separates and collects recyclables using a webcam and You Only Look Once (YOLO) real-time object detection in Raspberry Pi, to detect and classify these recyclables into their correct categories. The cloud IoT analytics analyze the solar e-waste in a different locations in industries.The proposed system works better and provides accurate results by using machine learning approach. Real-time mobile app monitors the bin’s level and location. The system monitors the smart bin levels and sends the notifications to alert and initiate the collection unit. Delay is introduced in the order of 3–8 s while the alert message is sent to the common waste collection unit. It helps to predict the level of the smart bin. The smart dust bin classifies the waste materials, and notifies its level to the collection center through the IoT platform when the level reaches a prescribed threshold, the signal corresponding to the level is passed to the common waste collection unit. The k-NN algorithm provides 83% accuracy in predicting the bin level in a real-time testing environment. It also helps in identifying the type of waste material. These algorithms are useful in updating the level of the bin via alert messages. The proposed smart bin uses k-Nearest Neighbor’s algorithm (k-NN) and Long Short-Term Memory (LSTM), a network-based learning algorithm. The smart bin with the Internet of Things (IoT) utilizes a machine learning approach to collect solar waste. This research paper focuses on the recycling process for solar PV modules using the Internet of Things in industries. The rapid increase in photovoltaic (PV) module installations provides a better energy conversion, but their life cycle is a major concern. Nowadays, modern industries generate their energy by using renewable solar.
